Bitget: #4 al mondo per volume di trading giornaliero!
Quota di mercato di BTC61.85%
Nuovi listing su Bitget : Pi Network
BTC/USDT$83524.96 (-1.36%)Indice Paura & Avidità25(Paura)
Indice Altcoin Season:0(Stagione di Bitcoin)
Monete listate nel Pre-MarketPAWS,WCTFlusso netto totale di ETF Spot su Bitcoin +$334M (1G); +$227.8M (7G).Pacchetto regalo di benvenuto per i nuovi utenti del valore di 6.200 USDT.Riscatta
Fai trading sempre e ovunque con l'app Bitget. Scarica ora
Bitget: #4 al mondo per volume di trading giornaliero!
Quota di mercato di BTC61.85%
Nuovi listing su Bitget : Pi Network
BTC/USDT$83524.96 (-1.36%)Indice Paura & Avidità25(Paura)
Indice Altcoin Season:0(Stagione di Bitcoin)
Monete listate nel Pre-MarketPAWS,WCTFlusso netto totale di ETF Spot su Bitcoin +$334M (1G); +$227.8M (7G).Pacchetto regalo di benvenuto per i nuovi utenti del valore di 6.200 USDT.Riscatta
Fai trading sempre e ovunque con l'app Bitget. Scarica ora
Bitget: #4 al mondo per volume di trading giornaliero!
Quota di mercato di BTC61.85%
Nuovi listing su Bitget : Pi Network
BTC/USDT$83524.96 (-1.36%)Indice Paura & Avidità25(Paura)
Indice Altcoin Season:0(Stagione di Bitcoin)
Monete listate nel Pre-MarketPAWS,WCTFlusso netto totale di ETF Spot su Bitcoin +$334M (1G); +$227.8M (7G).Pacchetto regalo di benvenuto per i nuovi utenti del valore di 6.200 USDT.Riscatta
Fai trading sempre e ovunque con l'app Bitget. Scarica ora
In relazione alle monete
Calcolatore dei prezzi
Storico dei prezzi
Previsione del prezzo
Analisi tecnica
Guida all’acquisto di monete
Categoria di crypto
Calcolatore di profitto

Prezzo di GrokXAI
Non listato
Valuta di quotazione:
EUR
I dati provengono da fornitori di terze parti. Questa pagina e le informazioni fornite non supportano alcuna criptovaluta specifica. Vuoi fare trading con le monete listate? Clicca qui
€0.001974-4.92%1D
Grafico dei prezzi
TradingView
Ultimo aggiornamento il 2025-04-03 02:43:17(UTC+0)
Capitalizzazione di mercato:--
Capitalizzazione di mercato completamente diluita:--
Volume (24h):--
Volume 24h / Cap. di mercato:0.00%
Massimo di 24h:€0.002108
Minimo di 24h:€0.001867
Massimo storico:€0.02747
Minimo storico:€0.001832
Offerta circolante:-- XAI
Offerta totale:
--XAI
Tasso di circolazione:0.00%
Offerta massima:
--XAI
Prezzo in BTC:-- BTC
Prezzo in ETH:39,308.95 ETH
Prezzo con la capitalizzazione di mercato di BTC:
--
Prezzo con capitalizzazione di mercato di ETH:
--
Contratti:
0x0009...605ae5d(BNB Smart Chain (BEP20))
Come ti senti oggi in merito a Grok?
Nota: queste informazioni sono solo di riferimento.
Prezzo di Grok oggi
Il prezzo attuale di Grok è €0.001974 per (XAI / EUR) oggi con una capitalizzazione di mercato aggiornata di -- EUR. Il volume di trading di 24 ore è €0.00 EUR. Il prezzo da XAI a EUR è aggiornato in tempo reale. Grok è -4.92% nelle ultime 24 ore. Ha un’offerta circolante di -- .
Qual è il prezzo più alto di XAI?
XAI ha un massimo storico (ATH) di €0.02747, registrato il .
Qual è il prezzo più basso di XAI?
XAI ha un minimo storico (ATL) di €0.001832, registrato il .
Previsione del prezzo di Grok
Quando è il momento giusto per acquistare XAI? Dovrei acquistare o vendere XAI ora?
Quando decidi se acquistare o vendere XAI, devi innanzitutto considerare la tua strategia di trading. L'attività di trading tra i trader a lungo e a breve termine sarà diversa. L'Analisi tecnica di XAI di Bitget può fornire un riferimento per il trading.
Secondo l'Analisi tecnica di XAI (4h), il segnale di trading è Acquista adesso.
Secondo l'Analisi tecnica di XAI (1d), il segnale di trading è Acquista adesso.
Secondo l'Analisi tecnica di XAI (1w), il segnale di trading è Acquista adesso.
Quale sarà il prezzo di XAI nel 2026?
In base al modello di previsione della performance storica del prezzo di XAI, si prevede che il prezzo di XAI raggiungerà quota €0.{8}1598 nel 2026.
Quale sarà il prezzo di XAI nel 2031?
Nel 2031, il prezzo di XAI dovrebbe aumentare del +23.00%. Entro la fine del 2031, si prevede che il prezzo di XAI raggiunga quota €0.{8}4751, con un ROI cumulativo del -100.00%.
Storico dei prezzi di Grok (EUR)
Il prezzo di Grok è variato di un -81.41% nell’ultimo anno. Il prezzo più alto di Grok in EUR nell’ultimo anno è stato €0.01862, mentre il prezzo più basso di Grok in EUR nell’ultimo anno è stato €0.001867.
DataVariazione del prezzo (%)
Prezzo più basso
Prezzo più alto 
24h-4.92%€0.001867€0.002108
7d-7.02%€0.001867€0.002152
30d-5.63%€0.001867€0.002152
90d-45.09%€0.001867€0.005495
1y-81.41%€0.001867€0.01862
Tutto il periodo+7.35%€0.001832(--, Oggi )€0.02747(--, Oggi )
Informazioni sul mercato Grok
Storico della capitalizzazione di mercato di Grok
Capitalizzazione di mercato
--
Capitalizzazione di mercato completamente diluita
--
Classifiche di mercato
Saldo di Grok per concentrazione
Whale
Investitori
Retail
Indirizzi Grok per durata dell'holding
Holder
Cruiser
Trader
Grafico del prezzo di coinInfo.name (12) in tempo reale
Valutazioni di Grok
Valutazioni medie della community
4.4
Questo contenuto è a puro scopo informativo.
XAI a valuta locale
1 XAI a MXN$0.041 XAI a GTQQ0.021 XAI a CLP$2.061 XAI a HNLL0.061 XAI a UGXSh7.861 XAI a ZARR0.041 XAI a TNDد.ت0.011 XAI a IQDع.د2.821 XAI a TWDNT$0.071 XAI a RSDдин.0.231 XAI a DOP$0.141 XAI a MYRRM0.011 XAI a GEL₾0.011 XAI a UYU$0.091 XAI a MADد.م.0.021 XAI a OMRر.ع.01 XAI a AZN₼01 XAI a KESSh0.281 XAI a SEKkr0.021 XAI a UAH₴0.09
- 1
- 2
- 3
- 4
- 5
Ultimo aggiornamento il 2025-04-03 02:43:17(UTC+0)
Notizie su Grok
Xai aggiorna le regole delle chiavi Sentry, riducendo il prezzo a 0,0143 ETH
Bitget•2024-12-12 09:51
Annuncio di Bitget sulla regolazione del decimale del prezzo minimo per 45 coppie di trading Spot
Bitget Announcement•2024-11-19 10:00
Musk: Piani per raddoppiare le dimensioni della startup di intelligenza artificiale XAI
Bitget•2024-10-29 14:38
Aethir e Xai lanciano un programma di finanziamento dell'ecosistema da 10 milioni di dollari
Bitget•2024-09-18 12:25
Nuovi listing su Bitget
Nuovi listing
Acquista di più
FAQ
Qual è il prezzo attuale di Grok?
Il prezzo in tempo reale di Grok è €0 per (XAI/EUR), con una capitalizzazione di mercato attuale di -- EUR. Il valore di Grok è soggetto a frequenti fluttuazioni a causa dell’attività continua, 24 ore su 24 e 7 giorni su 7, del mercato crypto. Il prezzo attuale di Grok in tempo reale e i suoi dati storici sono disponibili su Bitget.
Qual è il volume di trading di 24 ore di Grok?
Nelle ultime 24 ore, il volume di trading di Grok è --.
Qual è il massimo storico di Grok?
Il massimo storico di Grok è €0.02747. Questo massimo storico è il prezzo più alto di Grok da quando è stato lanciato.
Posso acquistare Grok su Bitget?
Sì, Grok è attualmente disponibile sull’exchange centralizzato di Bitget. Per altre informazioni dettagliate, consulta la guida su Come acquistare grok .
Posso ottenere un guadagno costante investendo in Grok?
Ovviamente Bitget fornisce un piattaforma di trading strategico, con trading bot intelligenti per automatizzare le operazioni e ottenere dei profitti.
Dove posso acquistare Grok con la commissione più bassa?
Siamo entusiasti di annunciare che la piattaforma di trading strategico è ora disponibile sull’exchange di Bitget. Bitget offre delle commissioni di trading e una profondità tra le migliori del settore per garantire ai trader investimenti redditizi.
Dove posso acquistare crypto?
Sezione video: verifica rapida e accesso rapido al trading
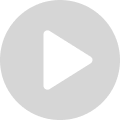
Come completare la verifica dell’identità su Bitget e proteggersi dalle frodi
1. Accedi al tuo account Bitget.
2. Se sei nuovo/a su Bitget, guarda il nostro tutorial su come creare un account.
3. Passa sull’icona del tuo profilo, clicca su “Non verificato” e quindi su “Verifica”.
4. Scegli il Paese o il territorio di emissione del tuo documento d’identità e il tipo di documento e segui le istruzioni.
5. Seleziona “Verifica mobile” o “Verifica PC” in base alle tue preferenze.
6. Inserisci i tuoi dati, invia una copia del tuo documento d’identità e scatta un selfie.
7. Invia la tua richiesta ed è fatta: hai completato la verifica dell’identità!
Gli investimenti in criptovalute, incluso l’acquisto di Grok online tramite Bitget, sono soggetti a rischio di mercato. Bitget ti fornisce modalità facili e pratiche per acquistare Grok. Ci impegniamo al massimo per informare gli utenti sulle criptovalute presenti sull’exchange. Ad ogni modo, non siamo responsabili per le conseguenze che si potrebbero verificare a seguito dell’acquisto di Grok. Questa pagine e le informazioni presenti non rappresentano un consiglio a investire su una determinata criptovaluta.
Bitget Insights
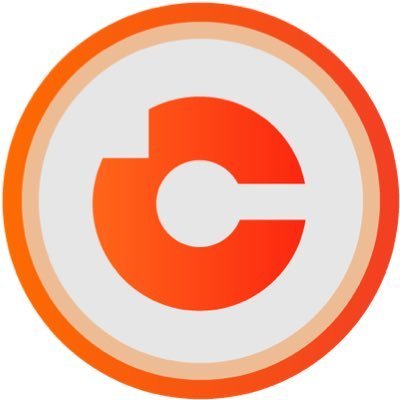
Cointribune EN
12h
Elon Musk Is Fighting For The Privacy Of Coinbase Users
Elon Musk, via his platform X, has filed a brief with the U.S. Supreme Court challenging the IRS’s practices regarding access to Coinbase user data. This initiative is part of a broader debate on privacy protection in the crypto space.
X Corp, Elon Musk’s company that manages the X platform, filed an amicus curiae brief with the U.S. Supreme Court on Friday, contesting the IRS’s methods.
The company specifically denounces the use of so-called “no-suspicion” subpoenas allowing the tax authorities to access, without a judicial warrant, the financial data of users on platforms like Coinbase.
The case highlights how the tax authorities obtained, through simple administrative subpoena, three years of transaction statements concerning over 14,000 Coinbase customers, including James Harper, the main plaintiff.
Alongside seven advocacy groups and researchers, X Corp denounces these “no-suspicion subpoenas” as a violation of the Fourth Amendment, which protects Americans against unreasonable searches.
Following this request, the Supreme Court asked the federal government on Monday to formulate an official response, highlighting the importance of this case. The dispute dates back to 2020 when James Harper sued the IRS to contest the seizure of his personal information related to cryptos.
In 2023, a federal court ruled in favor of the IRS, determining that the tax agency was acting within the scope of its legal prerogatives.
The current appeal before the Supreme Court thus marks a new stage in this legal battle, with potentially significant implications for the protection of digital financial data.
This initiative perfectly aligns with Elon Musk’s vision regarding digital governance. The billionaire, who recently sold his platform X to his own company xAI for 33 billion dollars, has always positioned himself as an advocate for privacy and freedom of speech.
By taking a stand for the protection of cryptocurrency users’ data, Musk strengthens his credibility among the tech and crypto communities, particularly sensitive to privacy issues.
The Supreme Court’s verdict could redefine the limits of state power in relation to digital privacy. This case resonates with the recent case of Tornado Cash , a crypto mixing protocol ultimately removed from the OFAC blacklist after a court ruled that the agency had overstepped its authority.
This case resonates with the recent case of Tornado Cash , a crypto mixing protocol ultimately removed from the OFAC blacklist after a court ruled that the agency had overstepped its authority, illustrating the growing tensions between state regulation and digital freedoms.
XAI+3.73%
ELON+2.87%
Mahnoor-Baloch007
1g
AI agents and AI are related but distinct concepts in the field of artificial intelligence.
AI (Artificial Intelligence)
1. Definition: AI refers to the broad field of study focused on creating intelligent machines that can perform tasks that typically require human intelligence.
2. Characteristics: AI systems can process and analyze large amounts of data, learn from experiences, and make decisions based on that data.
3. Examples: AI-powered chatbots, image recognition systems, and natural language processing tools.
AI Agents
1. Definition: AI agents are a specific type of AI system that can autonomously perform tasks on behalf of a user or another system.
2. Characteristics: AI agents have the ability to design their own workflow, utilize available tools, and interact with external environments to achieve complex goals.
3. Examples: AI-powered trading bots, autonomous vehicles, and smart home systems.
Key Differences
1. Autonomy: AI agents have a higher level of autonomy compared to traditional AI systems, allowing them to make decisions and take actions independently.
2. Interactivity: AI agents can interact with their environment and other systems, whereas traditional AI systems may only process data internally.
3. Proactivity: AI agents can anticipate and prevent problems, whereas traditional AI systems may only react to problems after they occur.
4. Complexity: AI agents often require more complex decision-making and problem-solving capabilities compared to traditional AI systems.
In summary, while AI refers to the broader field of artificial intelligence, AI agents are a specific type of AI system that can autonomously perform tasks, interact with their environment, and make decisions independently.
Thank you...🙂
$BTC $ETH $SOL $PI $XRP $DOGE $SHIB $SUNDOG $MEME $AI $XAI $PEPECOIN $PIPPIN $ORAI $ETC $WHY $U2U
SUNDOG+2.01%
BTC+1.23%
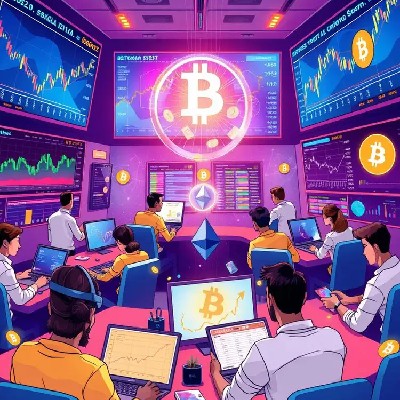
Crypto_inside
1g
Machine learning ❌ Traditional learning. 🧐😵💫
Machine learning and traditional learning are two distinct approaches to learning and problem-solving.
Traditional Learning:
1. Rule-based: Traditional learning involves explicit programming and rule-based systems.
2. Human expertise: Traditional learning relies on human expertise and manual feature engineering.
3. Fixed models: Traditional learning uses fixed models that are not updated automatically.
Machine Learning:
1. Data-driven: Machine learning involves learning from data and improving over time.
2. Algorithmic: Machine learning relies on algorithms that can learn from data and make predictions.
3. Adaptive models: Machine learning uses adaptive models that can update automatically based on new data.
Key Differences:
1. Learning style: Traditional learning is rule-based, while machine learning is data-driven.
2. Scalability: Machine learning can handle large datasets and complex problems, while traditional learning is limited by human expertise.
3. Accuracy: Machine learning can achieve higher accuracy than traditional learning, especially in complex domains.
Advantages of Machine Learning:
1. Improved accuracy: Machine learning can achieve higher accuracy than traditional learning.
2. Increased efficiency: Machine learning can automate many tasks, freeing up human experts for more complex tasks.
3. Scalability: Machine learning can handle large datasets and complex problems.
Disadvantages of Machine Learning:
1. Data quality: Machine learning requires high-quality data to learn effectively.
2. Interpretability: Machine learning models can be difficult to interpret and understand.
3. Bias: Machine learning models can perpetuate biases present in the training data.
When to Use Machine Learning:
1. Complex problems: Machine learning is well-suited for complex problems that require pattern recognition and prediction.
2. Large datasets: Machine learning can handle large datasets and identify trends and patterns.
3. Automating tasks: Machine learning can automate many tasks, freeing up human experts for more complex tasks.
When to Use Traditional Learning:
1. Simple problems: Traditional learning is well-suited for simple problems that require explicit programming and rule-based systems.
2. Small datasets: Traditional learning is suitable for small datasets where machine learning may not be effective.
3. Human expertise: Traditional learning relies on human expertise and manual feature engineering, making it suitable for domains where human expertise is essential.
Thank you...🙂
$BTC $ETH $SOL $PI $AI $XAI $BGB $BNB $DOGE $DOGS $SHIB $BONK $MEME $XRP $ADA $U2U $WUF $PARTI $WHY
BTC+1.23%
BGB+1.24%
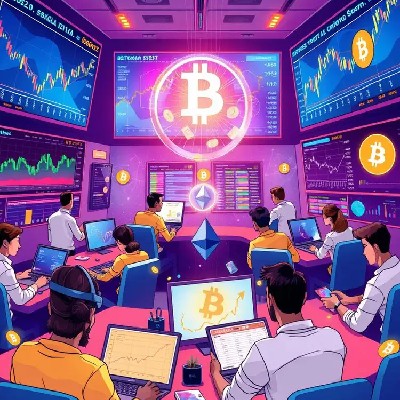
Crypto_inside
1g
What is Q-learning...🤔🤔??
Q-learning is a type of reinforcement learning algorithm used in machine learning and artificial intelligence. It's a model-free, off-policy learning algorithm that helps agents learn to make decisions in complex, uncertain environments.
Key Components:
1. Agent: The decision-maker that interacts with the environment.
2. Environment: The external system with which the agent interacts.
3. Actions: The decisions made by the agent.
4. Rewards: The feedback received by the agent for its actions.
5. Q-function: A mapping from states and actions to expected rewards.
How Q-learning Works:
1. Initialization: The agent starts with an arbitrary Q-function.
2. Exploration: The agent selects an action and observes the resulting state and reward.
3. Update: The agent updates its Q-function based on the observed reward and the expected reward for the next state.
4. Exploitation: The agent chooses the action with the highest Q-value for the current state.
Advantages:
1. Simple to implement: Q-learning is a straightforward algorithm to understand and code.
2. Effective in complex environments: Q-learning can handle complex, dynamic environments with many states and actions.
Disadvantages:
1. Slow convergence: Q-learning can require many iterations to converge to an optimal policy.
2. Sensitive to hyperparameters: The performance of Q-learning is highly dependent on the choice of hyperparameters.
Q-learning is a powerful algorithm for reinforcement learning, but it can be challenging to tune and may not always converge to an optimal solution.
Thank you...🙂
$BTC $ETH $SOL $PI $AI $XAI $XRP $BGB $BNB $DOGE $DOGS $SHIB $BONK $FLOKI $U2U $WUF $WHY $SUNDOG $COQ $PEPE
SUNDOG+2.01%
BTC+1.23%
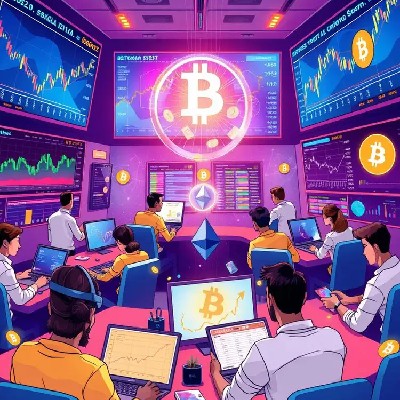
Crypto_inside
1g
What is Machine learning..🤔🤔??
Machine learning is a subset of artificial intelligence (AI) that involves training algorithms to learn from data and make predictions, decisions, or recommendations without being explicitly programmed.
Key Characteristics:
1. Learning from data: Machine learning algorithms learn patterns and relationships in data.
2. Improving over time: Machine learning models improve their performance as they receive more data.
3. Making predictions or decisions: Machine learning models make predictions, decisions, or recommendations based on the learned patterns.
Types of Machine Learning:
1. Supervised Learning: The algorithm learns from labeled data to make predictions.
2. Unsupervised Learning: The algorithm learns from unlabeled data to identify patterns.
3. Reinforcement Learning: The algorithm learns through trial and error to achieve a goal.
4. Semi-supervised Learning: The algorithm learns from a combination of labeled and unlabeled data.
5. Deep Learning: A subset of machine learning that uses neural networks with multiple layers.
Machine Learning Applications:
1. Image Recognition: Image classification, object detection, and facial recognition.
2. Natural Language Processing (NLP): Text classification, sentiment analysis, and language translation.
3. Speech Recognition: Speech-to-text and voice recognition.
4. Predictive Analytics: Forecasting, regression, and decision-making.
5. Recommendation Systems: Personalized product recommendations.
Machine Learning Algorithms:
1. Linear Regression: Linear models for regression tasks.
2. Decision Trees: Tree-based models for classification and regression.
3. Random Forest: Ensemble learning for classification and regression.
4. Support Vector Machines (SVMs): Linear and non-linear models for classification and regression.
5. Neural Networks: Deep learning models for complex tasks.
Machine Learning Tools and Frameworks:
1. TensorFlow: Open-source deep learning framework.
2. PyTorch: Open-source deep learning framework.
3. Scikit-learn: Open-source machine learning library.
4. Keras: High-level neural networks API.
Machine learning has numerous applications across industries, including healthcare, finance, marketing, and more. Its ability to learn from data and improve over time makes it a powerful tool for solving complex problems.
Thank you...🙂
$BTC $ETH $SOL $PI $AI $XAI $BGB $BNB $DOGE $SHIB $FLOKI $BONK $U2U $WUF $WHY $SUNDOG $PARTI $XRP
SUNDOG+2.01%
BTC+1.23%
Asset correlati
Criptovalute popolari
Una selezione delle 8 crypto con il più alto market cap.
Aggiunte di recente
Le criptovalute aggiunte più di recente.
Cap. di mercato comparabile
Tra tutti gli asset su Bitget, questi 8 sono i più vicini a Grok in termini di market cap.
